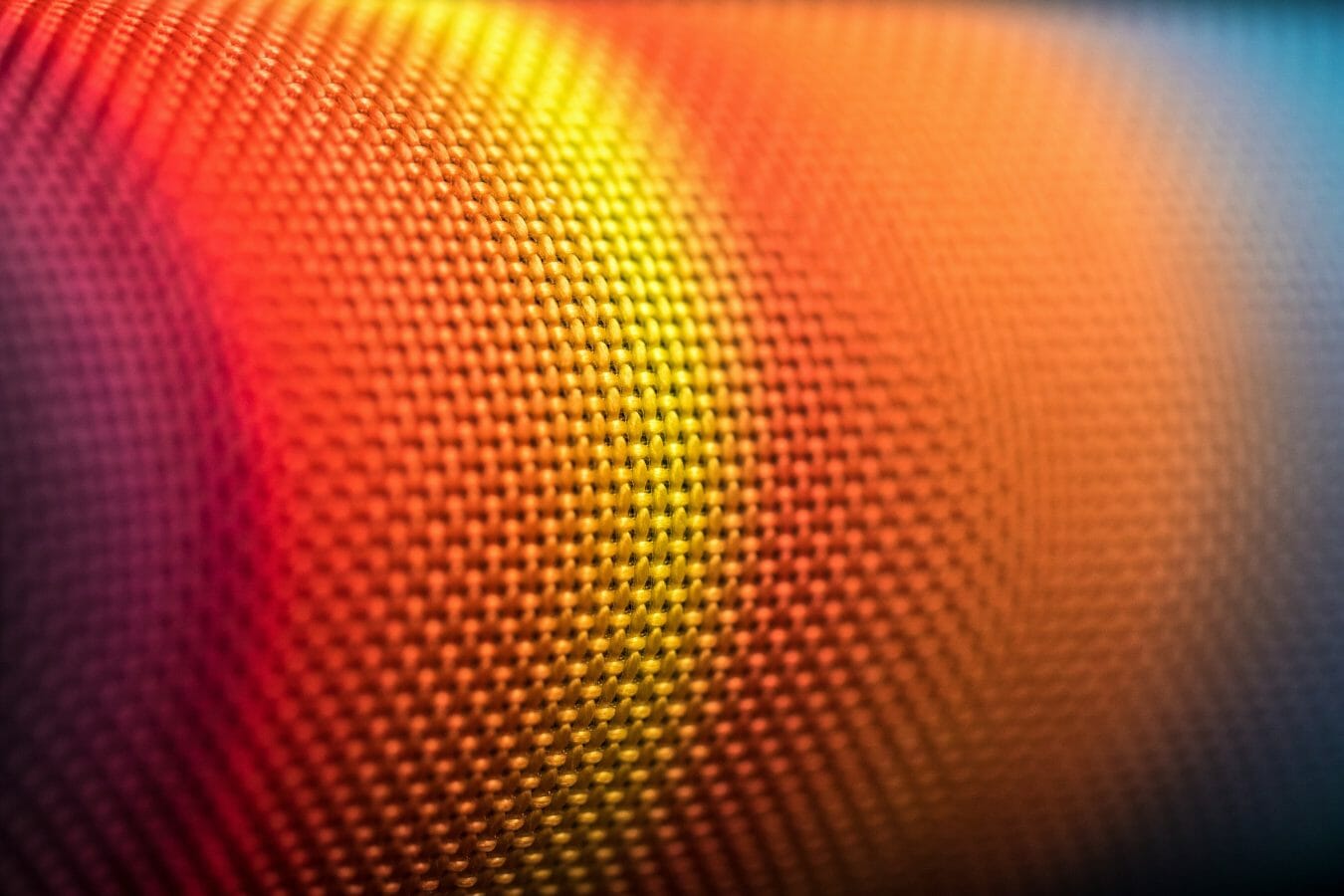
Articles
Industry News
Rising Concerns About Algorithmic Decision Making
By Cait Etherington
December 13, 2018
A new PEW Research Center study has found that most Americans don’t trust the algorithms that now being adopted to guide decisions about their lives. But will low trust for algorithmic decision making slow the pace at which machine learning is adopted, especially in schools and higher education?
Pessimism Prevails in the Face of Algorithmic Decision Making
A summary of the PEW Research Center’s new study suggests that with few exceptions, the public views algorithmic decision making as unfair. To reach this conclusion, PEW asked respondents their opinion on four different adaptive algorithm scenarios. They included: “a personal finance score used to offer consumers deals or discounts; a criminal risk assessment of people up for parole; an automated resume screening program for job applicants; and a computer-based analysis of job interviews,” according to PEW.
The research center found that, “Only around one-third of Americans think that the video job interview and personal finance score algorithms would be fair to job applicants and consumers.” In fact, the survey discovered, “Two-thirds of Americans (68%) find the personal finance score algorithm unacceptable, and 67% say the computer-aided video job analysis algorithm is unacceptable.”
Somewhat surprisingly, Americans seem to have a wide range of reasons for concluding that algorithmic decision making is unfair. While some of the participants in the PEW survey worried that algorithms are violating privacy rights, other respondents had more esoteric reasons for worrying about the growing presence of algorithms in their lives. Among those surveyed, 36% expressed concern that algorithms “remove the human element from important decisions.” A smaller percentage emphasized that humans are complex beings and algorithms are incapable of capturing such nuance.
Education and Algorithmic Decision Making
If the majority of those people surveyed in the PEW Research Center study are right and algorithms actually are biased, the implications for edtech could be significant. This is largely due to the fact that edtech increasingly relies on machine learning, which is all about algorithmic forms of decision making.
In the case of machine learning, algorithms essentially enable a machine or program to “learn” from a set of data, and this, in turn, enables the machine or program to solve new problems with little or no human intervention. In edtech, there is a lot of enthusiasm for machine learning because in some cases, it just makes more sense to rely on a machine than a human.
As an example, consider NUADU. This personalized learning platform relies on existing data to help make smarter decisions about which types of exercises and activities a student should attempt next in their quest to master new skills. A human teacher may also be able to make similar types of decisions, but there is one notable difference between the average human teacher and NUADU’s platform. While human teachers generally make decisions based on small samples (e.g., a few hundred students) and often rely heavily on anecdotal information, NUADU’s platform is able to compare vast amounts of data before recommending what types of assignments a student should tackle next. As Marcin Krasowski, COO VP of NUADU, explains, “The app learns student behavior, patterns, errors, answers et cetera. Based on this information, we are able to build a digital profile of each student and offer personalized learning resources and design unique learning paths.”
While this may sound ideal, there is one problem: Sometimes even data are biased.
Algorithmic Decisions and Algorithmic Biases
While there is certainly no indication that we should dismiss algorithmic decision making, over the past two years, the problem of algorithmic bias has gained growing attention. A 2017 article in the MIT Technology Review underscores the depth of the current problem:
A key challenge … is that crucial stakeholders, including the companies that develop and apply machine learning systems and government regulators, show little interest in monitoring and limiting algorithmic bias. Financial and technology companies use all sorts of mathematical models and aren’t transparent about how they operate …
While there is already an industry emerging to address algorithmic bias (e.g., companies like ORCCA help businesses that rely on algorithms critically assess where their algorithms may be reproducing biases), the recent PEW Research Center survey seems to suggest that we haven’t yet done enough to quell growing public fears that algorithms ultimately aren’t on our side. Of course, only time will tell whether these fears slow the pace at which machine learning is embraced, specifically in the education and training sectors.
Featured Image: Jason Leung, Unsplash.
No Comments