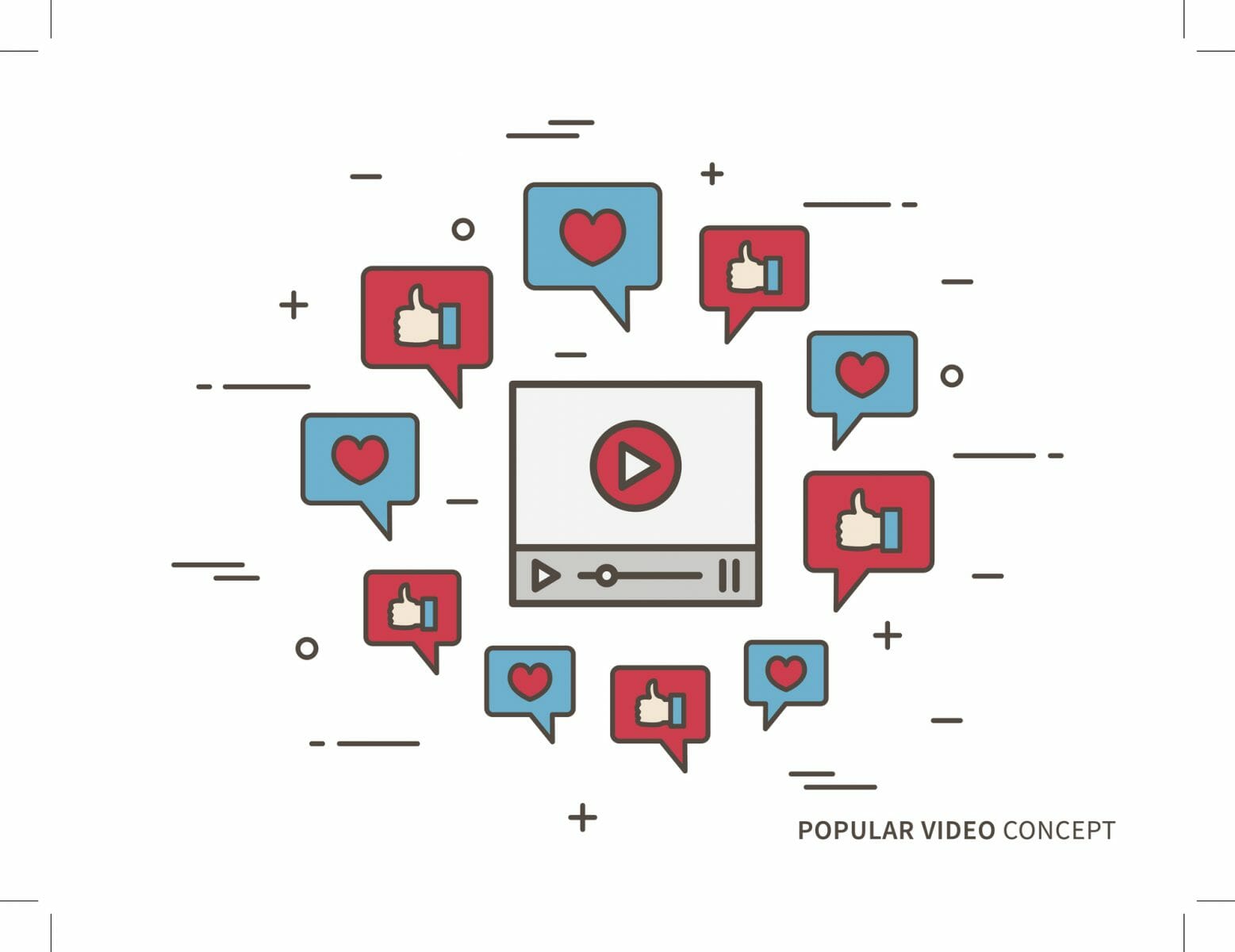
Interviews
Video Streaming and Transforming the Classroom: an Interview with Scott Martin, Co-Founder of Scriyb
By Henry Kronk
November 06, 2017
Professor Scott Martin previously served as the director of the Computer Game Design Program at George Mason University and still teaches there as well. He is also the founding director of the institutions Virginia Serious Game Institute.
As an higher-ed instructor and administrator with 30 years’ experience, Martin was keen to move courses online, but met opposition at several levels.
“All I heard were groans from my faculty when online education discussions arose,” Martin told me. “Legacy LMS platforms create more work: more emails, VMs, longer office hours, more critical student evaluations.”
“’I’ll only teach online if you let me cap the course at 20 students,’ [some professors said] which somewhat defeats the purposes of scaling courses to a greater number of students. We’ve all heard the horror stories about the 60-student online class.”
In response, Martin and a team of developers created Scriyb, a live, interactive video streaming platform designed for teaching large classes.
The platform allows for two-way interaction between teacher and student. Also, with their machine-learning algorithm, dubbed Deep Academic Learinging Intelligence (DALI), Scriyb breaks up large student groups into manageable and more social small groups.
Scott Martin: We are all aware of the technical glitches and ‘coherence confusion’ when 35+ people logon to a chat room or AV platform, and the high student attrition numbers from MOOCS, which are really just (live) streaming broadcasts with little student real-time interactivity. Scriyb uses patented algorithms that segment large numbers of students into smaller learning cohorts, formed by academic achievement level and commonalities and interest, and walls them off from every other cohort.
Each cohort has their own communication channels to interact with each other in real-time during the class. This methodology not only allows a single teacher to live stream to hundreds and hundreds of students, but the mathematical grouping of cohorts also fosters true organic social engagement and peer-to-peer learning. It’s the scaling of teaching and learning holy grail!
Moreover, Scriyb’s Deep Academic Learning Intelligence (DALI) machine-learning models parse all the classroom discussions and banter, both subject matter discussions, and the equally important non-subject matter social exchanges. These datasets are used to provide personal AI-driven intervention methods like academic advising and counseling. The overall amount of generated learning science and assessment data is unprecedented.
Henry Kronk: So, teaching with live, interactive streaming can create pandemonium. In answer to this, DALI breaks up students into small groups. It seems that this would be great for the students, but does this help the professor access students or vice versa?
SM: Yes, within a large course chat or A/V channel incoherence occurs.
Some learners may ask pertinent questions, some adding semi-relevant commentary, some contributing drivel and irrelevant emojis, on top of additional text responses from the instructor, add a misplaced chatbot prompt, and a chat channel content coherence can quickly deteriorate. DALI’s patented algorithms groups learners into small cohorts to creates an ideal academic and social environment, and walls each group of learners from every other group. In essence, learners don’t know they may be in a course of 500 students, as each learner only communicates with their peers and the teacher. The grouping, or clustering algorithms variables are weighted to facilitate social engagement organically; to foster cohort/team/group learning organically.
An instructor manages a large Scriyb class as they would normally a traditional small onsite course: alternating lecture and questions, demonstrations and questions, in-class assignment, etc. The teacher can view all the chat channels activities, or just one or two at a time. She can provide an answer to a question verbally through live-streaming to the whole class, or to one group within the larger class. She can text an answer to one group, or even to one student in one group through private messaging. Additionally, in our internal studies, if the group characteristics are successfully composed, as in comingled achievement rates and the learners are socially complimentary, students answer other students’ questions 80% of the time, and the answers are correct 87% of the time, helping a teacher better manage large scale courses using the Scriyb methodology.
HK: So DALI matches up students of different levels to enhance the learning experience. Could you tell me in more detail how DALI determines the groups?
What philosophy/pedagogy went in to this matching method?
SM: DALI comingles 30% high achievers, 48% mid, and 22% low achiever (plus or minus 3%), within the same group, at the launch of an academic experience. The weights that define these levels of achievements obviously slides to accommodate any grading scale or system. This group composition has proven to increase academic performance for all the students in each group, as long as they have similar social commonalities, as mentioned before. Class composition between high, mid, and low achievers is critical to success: a blended formula of the peer-effect models found in the Boutique (students perform higher when surrounded by students similar to themselves), Shining Light (a single high achiever provides a great example for all other students), and the Rainbow peer-effect model (where an assortment of student abilities benefits the entire group) (Sacerdote, 2011). Caroline Hoxby and Gretchen Weingarth’s research paper, Taking Race Out of the Equation: School Reassignment and The Structure of Peer Effect (2006) discovered in their 20-year study at Wake County School District, North Carolina, that low-achievement students, appeared to greatly benefit academically from interacting with high-achievers with similar interests (personality characteristics, interest, commonalities) in the same classroom. Additionally, they also discovered that high achievers measurably benefited from studying alongside low achievers with the same interests and commonalities, (same type), demonstrating that efforts of combining students with disparate academic achievement levels in order to raise the academic threshold of an overall class, the students within a class must “…. maintain continuity of types” (Hoxby & Weingarth, 2006).
HK: A recent study released by Gallup and Inside Higher Ed found that 7 of 10 teachers who have taught online courses have learned new teaching skills and improved their instruction. Do you anticipate that the use of Scriyb will have this effect?
SM: Without a doubt, as all the class lectures and subsequent student’s synched chat questions are automatically archived every session. Therefore, we’ve had faculty view the archived version of their classes for pedagogical assessment, and discovered that the same section of their lectures kept generating questions, and similar questions as well. So, they revised their lectures and improved their content delivery for the next semester.
Moreover, DALI provides unprecedented datasets to determine if course learning goals and objectives outlined in a syllabus are being met, and where they may be faltering. DALI uses deep NLP (Neuro-linguistic programming) models to parse, tag and index every chat phrase, and determines if it is subject or non-subject matter chat, parses all communication between teacher/learner and learner/teacher, and matrixes all these datasets against a learner’s academic achievement level. If a learner’s academic performance is slipping, DALI will most likely know why, and can recommend an adaptive tutor, maybe an additional textbook, or even AI-driven TA assistance, all to help guide the learner toward academic success! It’s pretty remarkable actually.
HK: How much bandwidth does Scriyb take up? Let’s say an institution wanted to teach a course via Scriyb to 500 students – would that present an issue?
SM: Not really. We’re currently exploring a few partnerships to help launch academic programs in several emerging world countries. For the learner, Scriyb only requires access to Wi-Fi with 100Kbp/s download speed. Even G2 (Time Division Multiple Access) on a mobile phone would suffice. For the instructor or content provider, Ethernet would be ideal with bandwidth of 1-1.5Mbp/s. Depending on bandwidth issues, even in some U.S. public schools, we have used a hybrid model whereby students in a physical classroom all watched one projected teacher live-stream, and students used laptops to chat/text interact during class. Subject expert and off-site teachers were streamed into the class from all over the region. It was quite effective.
Professor Martin cited the following works in his answers to the above questions:
Hoxby, C., & Weingarth, G. (2006). Taking Race Out of the Equation: School Reassignment and the Structure of Peer Effect. Working Paper
Sacerdote, B. (2011). Peer Effect in Education: How Might They Work, How Big Are They, and How Much Do We Know Thus Far? In Handbook of the Economic of Education (pp. 249-273). Amsterdam, North-Holland.
4 Comments