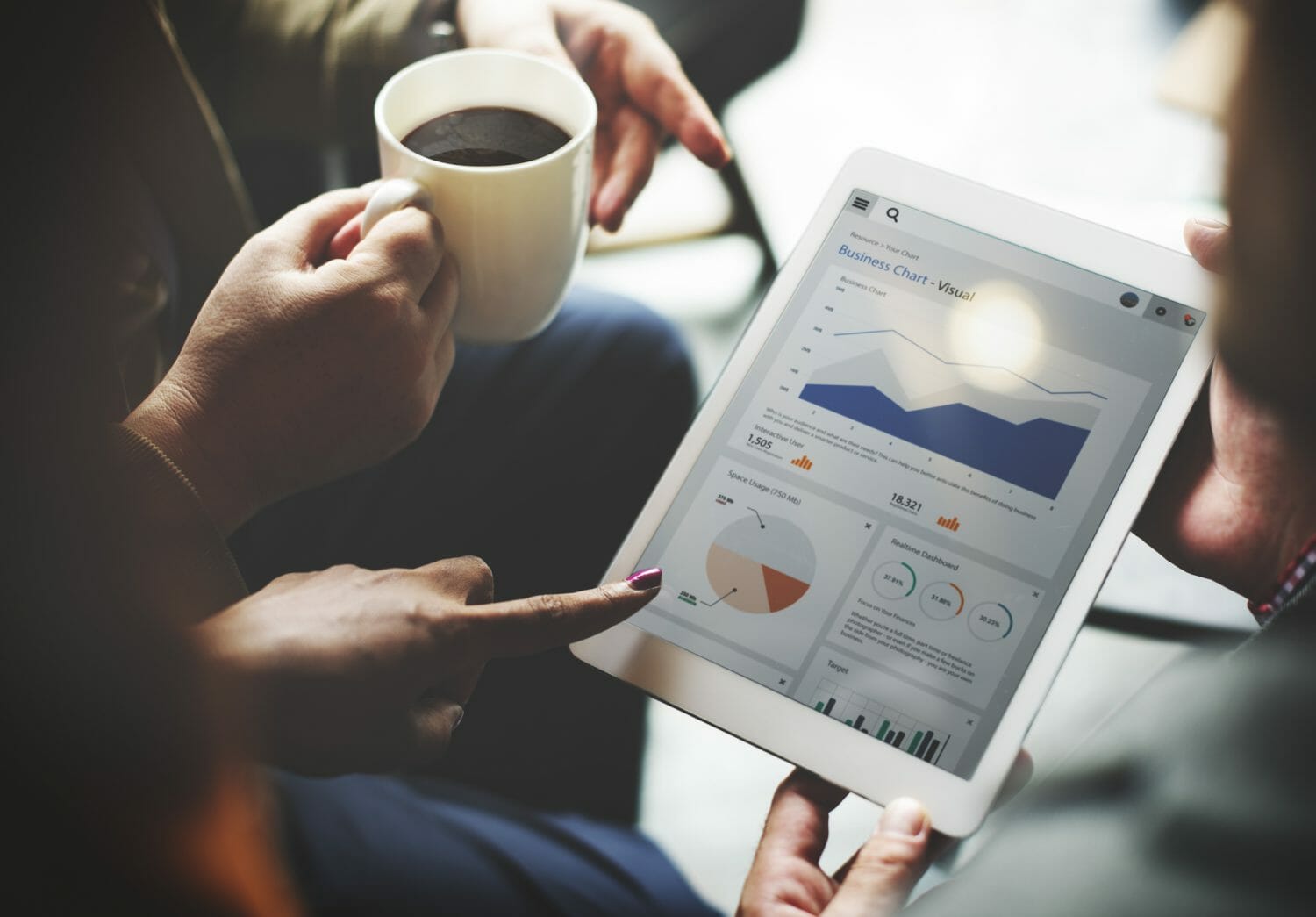
Articles
Industry News
Learning Analytics – Making Sense of the Data
By Ben Henderson
September 12, 2017
Learning analytics have become a hot topic within the e-Learning industry over the last couple of years thanks to more sophisticated ways of tracking and reporting learners. Organizations are now subscribing to the idea that creating content is just one part of training and developing individuals and that making sense of learning data is a necessary and valuable process. Big (and small) data is regularly mentioned in conjunction with user information and it certainly sounds impressive, but what is it? And more importantly how do organizations use it to their advantage and make the whole learning and training experience more effective? Even the term analytics can sound a little scary for training departments who have previously relied on feedback forms and formal assessments as measuring tools. There is no need to worry though, these terms might seem intimidating on the surface, but in reality, they are actually really helpful in defining modern day learning data and more importantly, making sense of it.
First of all, how can you measure learner progression in a way that works for you and your organization? Answering this question depends on how robust and sophisticated your tracking system is and how much time you would like to spend analyzing and sorting through the (potentially meaningless) data. Thanks to technology enabled analysis tools, a lot of this data can be analyzed in an automated fashion, meaning the more learners who participate, the more meaningful the data analytics. Any of the big MOOC companies (Coursera/FutureLearn etc) can rely on millions of learner records to generate valuable analysis of how individuals interact with digital courses, giving a fantastic insight into online learning behaviors from an individual and community perspective. Alongside this, the course itself can be analyzed in regards to design and accessibility, as well as how successful it has been in disseminating the content and organizing the group in real time. No longer do learning providers have to wait for a cohort to complete the course before changes are made to the design and structure. This can be done also in real time so current students receive these design improvements, rather than just the future cohorts.
Like most personal data, it is important that learners have agreed to share it and also know it will be analyzed as part of the learning process. Also, the data collected should be valuable and mean something to the organization and course creators; otherwise it’s a waste of time sharing in the first place. This analyzed information can be shared with course leaders in an easily accessible and clear manner, usually through a dashboard displaying visual representations of learner’s progress. Alongside this, the students themselves may also have access to their learning information, as well as how they fit in with the rest of the community. In a more traditional face to face learning environment, this analysis would be done in person on a regular basis through observation and in-class discussion, and a lot of it would be guesswork rather than detailed analytics.
Learning analytics certainly has a place in modern day training and development to produce more in-depth discussion on learning environments (and the learners themselves) both on and offline. The design of a particular course could potentially have severe ramifications on its success and objectives if not structured in the best way for that specific demographic of learners. Learning analytics is now allowing course creators and leaders to structure their content and activities in the most effective way to support learning outcomes, and in the process, getting the most out these learning environments. As these analytic tools advance, expect to see them become a regular part of the learning and development process to provide an insight like we’ve never seen before.
[…] electronic trends. For the entrepreneur looking at these successes and hoping to find their own niche in the digital tech market, it’s important to know that there’s more to being successful than just having a good […]
[…] electronic trends. For the entrepreneur looking at these successes and hoping to find their own niche in the digital tech market, it’s important to know that there’s more to being successful than just having a good […]