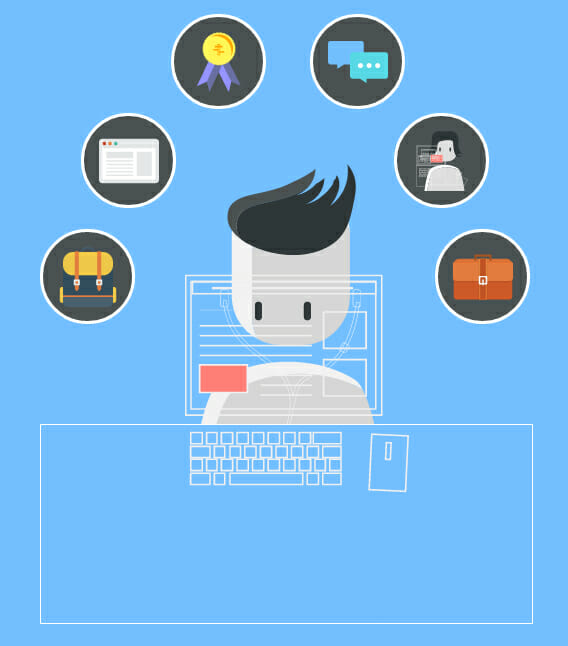
Articles
Editor’s Picks
Grading with AI: How Kadenze Powers Its Online Fine Arts Courses
By Henry Kronk
March 09, 2018
MOOCs, online courses, and other eLeraning initiatives have increased access to education for learners around the world. But there’s a kind of unspoken belief generally held in regard to online education: it works well with some subjects, but less so with others. STEM subjects, which typically require mastery of specific, discrete subject matter, are very popular online. But when it comes to more subjective matters, like social and emotional intelligence, the arts, or the fine arts, many find the online setting to pale in comparison to its in-person equivalent.
But one company is changing that narrative. To fine art purists, Kadenze does the unthinkable. Not only does it offer art courses that span “Designing Synthesizer Sounds” to “Custom Handlettering”; it grades student work with artificial intelligence (AI). The company has designed over 100 bots that implement various AI techniques, such as deep learning and machine learning, to assess everything from a hand-drawn image to edited sound to film.
“Our graders can also analyse code, writing, and more traditional coursework. Our real special sauce is in assessing multimedia though,” said Jordan Hochenbaum, one of the chief architects of Kadenze’s AI grading bots.
I asked him a few questions about the Kadenze process.
Let’s focus specifically on hand-drawn images – what are the bots looking for/testing?
Hochenbaum: It really depends on the tasks/learning outcomes. In our Comics course for example, we’ve done some novel work in automatically detecting comic panels and the type of content within a panel (image, text). From there the machine can begin to understand the use of different panel layouts, transitions, and how they are used for narrative, etc. This would have very different goals than an AI grader that was aimed at detecting a specific kind of drawing technique, brush stroke, use of color, etc.
Isn’t there a good deal of bias that goes into creating an algorithm that can judge art?
In general these types of algorithms are trying to generalize about the things that they’ve seen as much as possible, so that when they see something new, they can provide an appropriate response. However, bias can be present for a number of reasons, like not having a large or diverse enough set of training data. But like humans, these algorithms can get better over time, as they see more examples and gain experience.
One thing to note however is that we typically use these types of machine grading for assessing more technical aspects of the work, and leave judging of subjective ideas or aesthetics to student participation in the coursework galleries.
Do the Kadenze bots also provide feedback?
Totally! Sometimes it’s written feedback related to a specific grading criteria or score. Other times we provide visual feedback which may not factor into the overall score or grading criteria, but is useful as a tool for the student to visualize some aspect of how they did or otherwise explore the work in a new way.
Is a human involved in any way in the grading process?
100%. Pretty much all of our “graders” and feedback tools are developed in an iterative process with the instructor and our R&D team. The grades might be benchmarked for consistency against how one or more instructors might grade that same assignment, or the feedback might come from a set of responses and language they actually use.
How do Kadenze learners respond to AI assessment?
In general as long as they are working people are happy. It’s also important though to really think about what kinds of tasks AI assessment is appropriate for. For example, in our course Sound Production in Ableton Live for Musicians and Artists, where students are learning to mix and produce music, the machine can actually provide a level of detail that is difficult for a human to replicate.
The computer can compare the student’s version of a mix (“Mix A”) against a reference mix (“Mix B”) of song, and say “Mix A is exactly 3.3dB (decibels) louder than Mix B, and Mix B has 6dB of more energy between 12kHz and 19kHz, and so it sound brighter.” For a human grader, we could probably just say “Mix A sounds a little louder than Mix B, and Mix B is brighter.”
For the more open-ended “creative” parts of the assignment though, things like aesthetics and compositional choices, it’s more appropriate to have the learners comment on each others work in the course gallery. This is aimed to give the same kind of experience students might have during “critique” in art school, or talking about their work over food with friends. By combining both approaches appropriately, we get the best of both worlds, and our learners generally respond well. Who knows though, maybe one day you’ll be engaging in a live discussion with a chat bot? 😉
No Comments