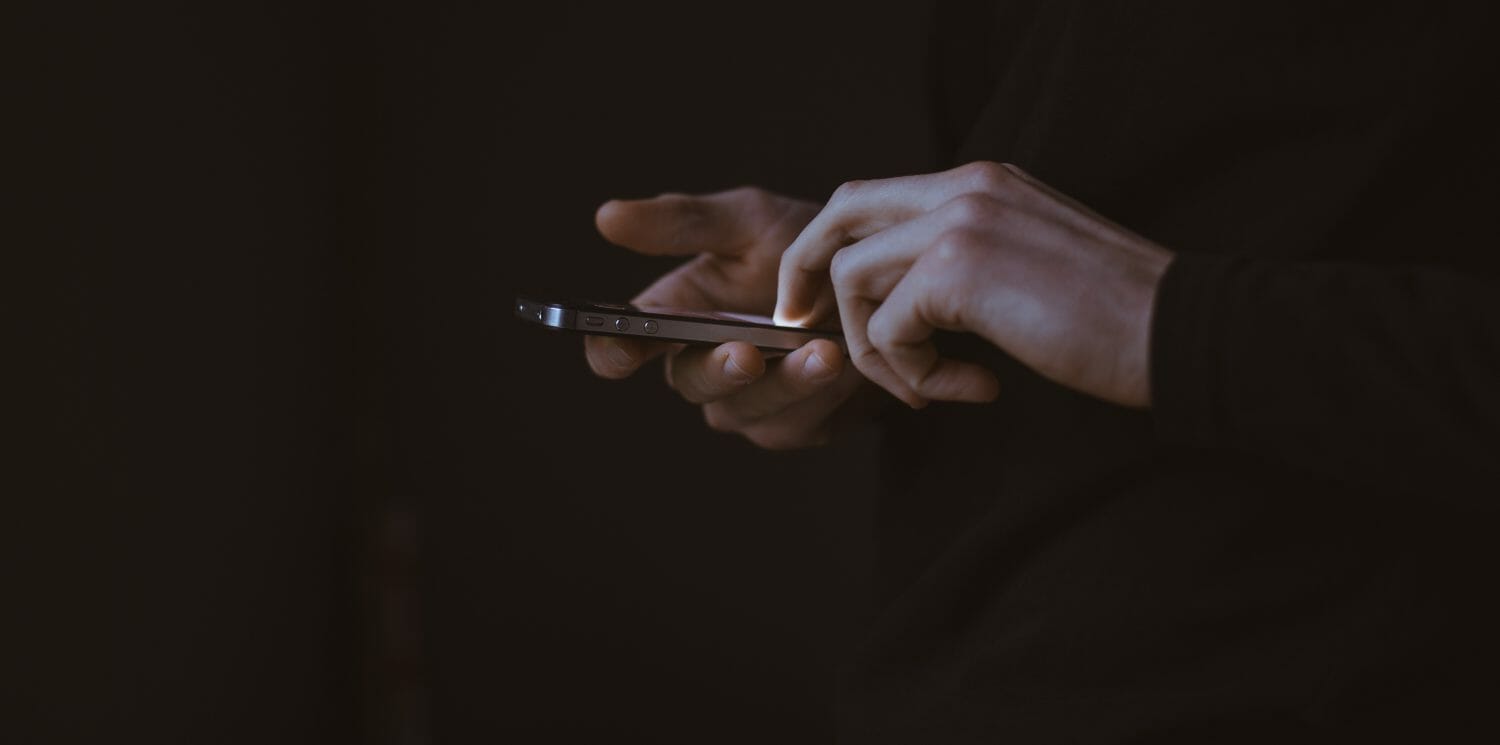
Big data is produced when we swipe in, use an ATM, do a Google search, and increasingly, even when we use a home appliance. In short, every digital process and social media exchange produces data, which means its volume and scope continues to expand. In the past, we were able to mine “structured data” (e.g., numbers in a spread sheet), but much of the other data we produce (e.g., when we post an update on Facebook or Twitter) was difficult to mine. It is now possible to mine both structured data and “unstructured data” (e.g., blog posts, articles and tweets), but we still face a challenge: How do we mine both structured and unstructured data in a manner that produces meaningful insights and results? This question is also critical when exploring the issue of big data and eLearning.
Big Data and eLearning
While the full impact of big data on eLearning is yet to be realized, there is no question that big data holds the potential to transform how virtual learning courses are developed, assessed and even taught. Moving forward both education and training will increasingly take place in virtual or blended environments. This means that learning activities themselves will generate a growing field of data. Data generated in online courses, however, can also in turn be mined to transform instructional design (e.g., by providing greater insight into how students learn). When big data and eLearning converge, there are many impacts. Three impacts that are already beginning to be realized include big data’s contribution to continuous assessment, geared-to-learner education, and virtual trainers and educators.
Continuous Assessment
In the past, learning and especially eLearning has tended to work on a learn-and-test model. Students watched a video and completed a quiz, or students finished a module and then carried out a formal assessment (e.g., a test). Big data applications enable us to constantly mine data, including unstructured data. As a result, it is now possible to assess learners on an ongoing basis. For example, rather than test a learner’s knowledge when they have concluded a module, one might assess their learning throughout the module by tracking their learning curve and then giving them a final grade based on the scale of their learning. In the past, this may have been possible but in reality, human teachers can only give so many test and work with so much data. Tracking a student’s understanding every minute and even every second of the class would be a challenging if not impossible endeavor. As the capacity to generate and mine both structured and unstructured data expands, it is becoming increasingly possible to develop courses that assess students from start to finish, and this in turn is opening up the possibility of assigning grades (where needed) based on learners’ progress rather than simply the standardized benchmarks they manage to reach. Indeed, this is one of the great promises of big data and eLearning.
Geared-to-Learner Education
Back in the 16th century, age-based grades did not exist. Learners of all ages came together to learn based on their level or ability alone. Over the past 500 years, learning had become increasingly focused on arbitrary factors, such as age, but this largely reflects the administrative limits of schools and workplaces to assess learners. Most public schools, for example, don’t possess the resources to assess every learner, place them at the right level, and then work with different levels of maturity in a single classroom or conversely create a separate and unique classroom for every learner with a dedicated teacher. Likewise, in the workplace, determining who does and does not require a specific set of skills is a time-consuming and costly endeavor. Bringing big data and eLearning together holds the potential to solve this problem by delivering only the education and training that specific learners require. Indeed, when big data and eLearning meet, it is possible to treat every learner as an individual with a specific set of learning or training needs. While this may not result in the end of age-based grades, it will likely profoundly transform workplace training in the near future and moving forward, it will ensure that fewer students and employees are asked to complete courses covering materials they have already mastered.
More Virtual Trainers and Educators
As recently explored in eLearningInside News‘ feature article on Jill Watson (a virtual teaching assistant at the Georgie Tech), students typically ask similar questions. As we continue to harness the ability to mine large fields of data, then, we will be able to more effectively predict and respond to learners’ questions and even develop courses bearing this knowledge in mind. This is exactly what Georgie Tech AI professor Ashok Goel is already exploiting. While Goel admits that his virtual TA got off to a rough start, by the end of the first semester, Jill Watson could respond to students’ questions with well over 90% accuracy. Jill Watson, of course, is one of the more intriguing and potentially impactful products of big data. Jill can not only respond to frequently asked questions but also be programmed to identify and refine the most effective responses. While virtual TAs, like Jill, will likely not displace real TAs, professors, teachers or trainers any time soon, in the world of big data, it seems likely that in the future, virtual trainers and educators will be added to human teaching teams. Notably, in the Georgia Tech experiment what ultimately gave Jill’s bot status away was not the quality of her answers but rather her working hours. Unlike Professor Goel and his human TAs, Jill would even respond to questions at 3:00. For this reason, data driven educational solutions may also finally solve one of education’s biggest challenges: Offering instruction to students when students do much of their work–in the middle of the night.
No Comments