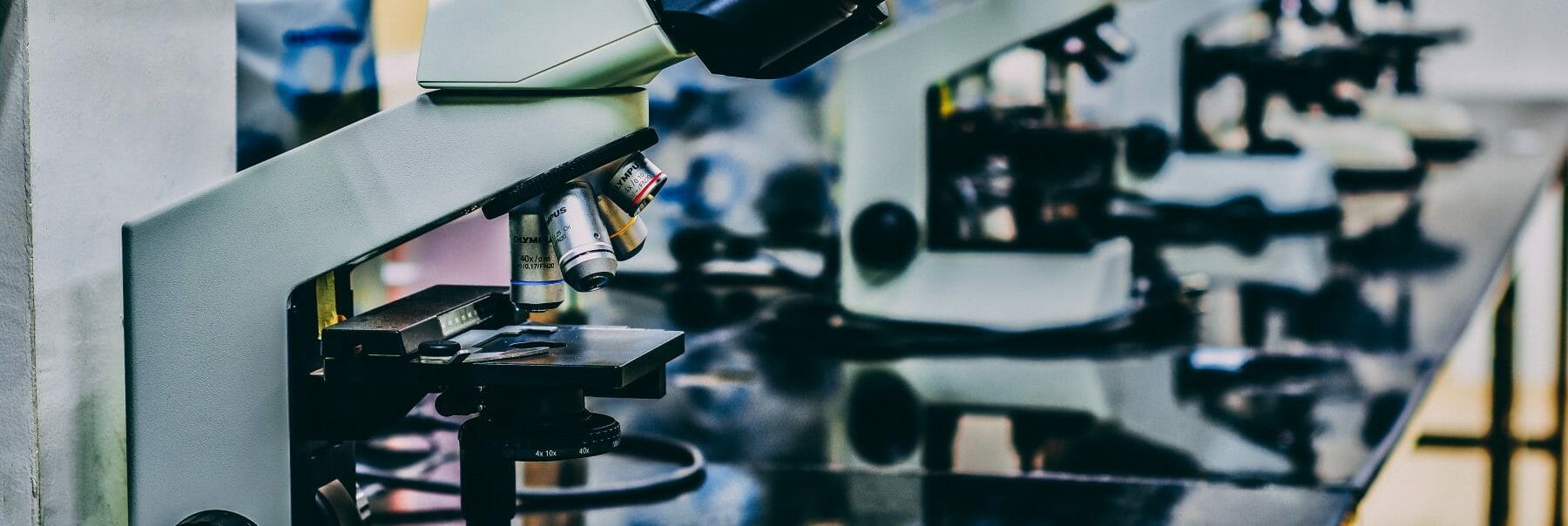
Articles
Editor’s Picks
K-12
An Adaptive Algorithm for K-12 Science Shows Promising Results
By Henry Kronk
January 14, 2019
Adaptive algorithms designed for the education process tend to generate mixed results. While applications teaching languages, math, and early literacy have shown promising results, other areas tend to be ill-suited for teacher bots. A team of Dutch education researchers, however, recently ventured into relatively unexplored terrain. Led by Karel Kroeze of the University of Twente, the team created an adaptive algorithm that would evaluate and aid learners in forming scientific hypotheses.
The Issue with K-12 Hypothesis Forming
In K-12 education, hypothesis forming can be a fraught process. While inquiry-based learning is generally regarded as a well-grounded pedagogy, researchers have identified issues in the inquiry-forming process. In other words, while students tend to learn well when they ask questions about a specific question, they can also struggle to ask a productive question in the first place.
As Kroeze et al. write, “Research has consistently shown that inquiry is a complex process in which students make mistakes. Specifically, students of all ages have problems in formulating hypotheses, particularly when they are unfamiliar with the topic of inquiry, and when experimental data is anomalous. As a consequence, few students generate hypotheses on their own account, and when they do, they often stick to a single hypothesis that is known to be true (i.e., confirmation bias) or formulate imprecise statements that cannot be tested in research. These natural tendencies demonstrate that unguided inquiry learning is likely to be ineffective.
“However, guided inquiry learning has been shown to compare favorably to both direct instruction and unguided inquiry learning, and helps foster a deeper conceptual understanding.”
The Adaptive Algorithm
Kroeze and his team set about to create an algorithm that could help guide students in the hypothesis creation process. To do this, the algorithm would need to do two things: 1) evaluate student hypotheses and 2) provide feedback on their quality.
To tackle task 1), the team referred to education researchers M.E. Quinn and K.D. George who, in a 1975 article, identified 5 qualities of a good hypothesis. These are:
“(1) it makes sense; (2) it is empirical, a (partial) scientific relation; (3) it is adequate, a scientific relation between at least two variables; (4) it is precise—a qualified and/or quantified relation; and (5) it states a test, an explicit statement of a test.”
Using a set of context-free grammar terms to define natural language (following the work of Noam Chomsky), the team created an algorithm for the online science platform Go-Lab. It integrated with the hypothesis scratchpad feature on the platform. The adaptive algorithm allowed students to use a set of pre-programmed terms such as ‘if,’ ‘then,’ ‘increases,’ ‘decreases,’ ‘is equal to,’ and so on to write their hypothesis. They were then given the option to form multiple hypotheses and, if they felt like it, to ask the algorithm for help.
The Experiment
The researchers then tested this algorithm in action in three different settings, creating two groups in each, with one being the test group and the other acting as the control.
“An initial pilot study was conducted with an early version of the hypothesis parser to assess the feasibility of automated parsing of hypotheses using a context-free grammar. Following that, a second pilot study was conducted with the complete version of the parser to identify any remaining issues with the parser and inquiry learning spaces (ILS) before moving on to the final experiment. The final experiment used a quasi-experimental design to assess the benefit of the tool in improving students’ hypotheses.”
The Results
Like many experiments, the researchers were able to produce positive results for their tool in a laboratory setting. Live in the classroom, their results were more mixed.
Still, when testing for the educational outcomes, learners using the algorithm who asked for help tended to score higher than their peers in the control group. As the authors conclude:
“An automated hypothesis scratchpad providing students with immediate feedback on the quality of their hypotheses was implemented using context-free grammars. The automated scratchpad was shown to be effective; students who used its feedback function created better hypotheses than those who did not. The use of context-free grammars makes it relatively straightforward to separate the basic syntax of hypotheses, language specific constructs, and domain specific implementations. This separation allows for the quick adaptation of the tool to new languages and domains, allowing configuration by teachers, and inclusion in a broad range of inquiry environments.”
These conclusions are exciting. They indicate that adaptive algorithms and personalized learning may have an important role to play in the future of science education.
Read the full study here.
Featured Image: Ousa Chea, Unsplash.
[…] On June 30, the private equity firm Carrick Capital Partners announced it had finalized plans to acquire the Flatiron School, a coding bootcamp, from WeWork. The Flatiron School offers training in software engineering, data science, and cybersecurity. […]