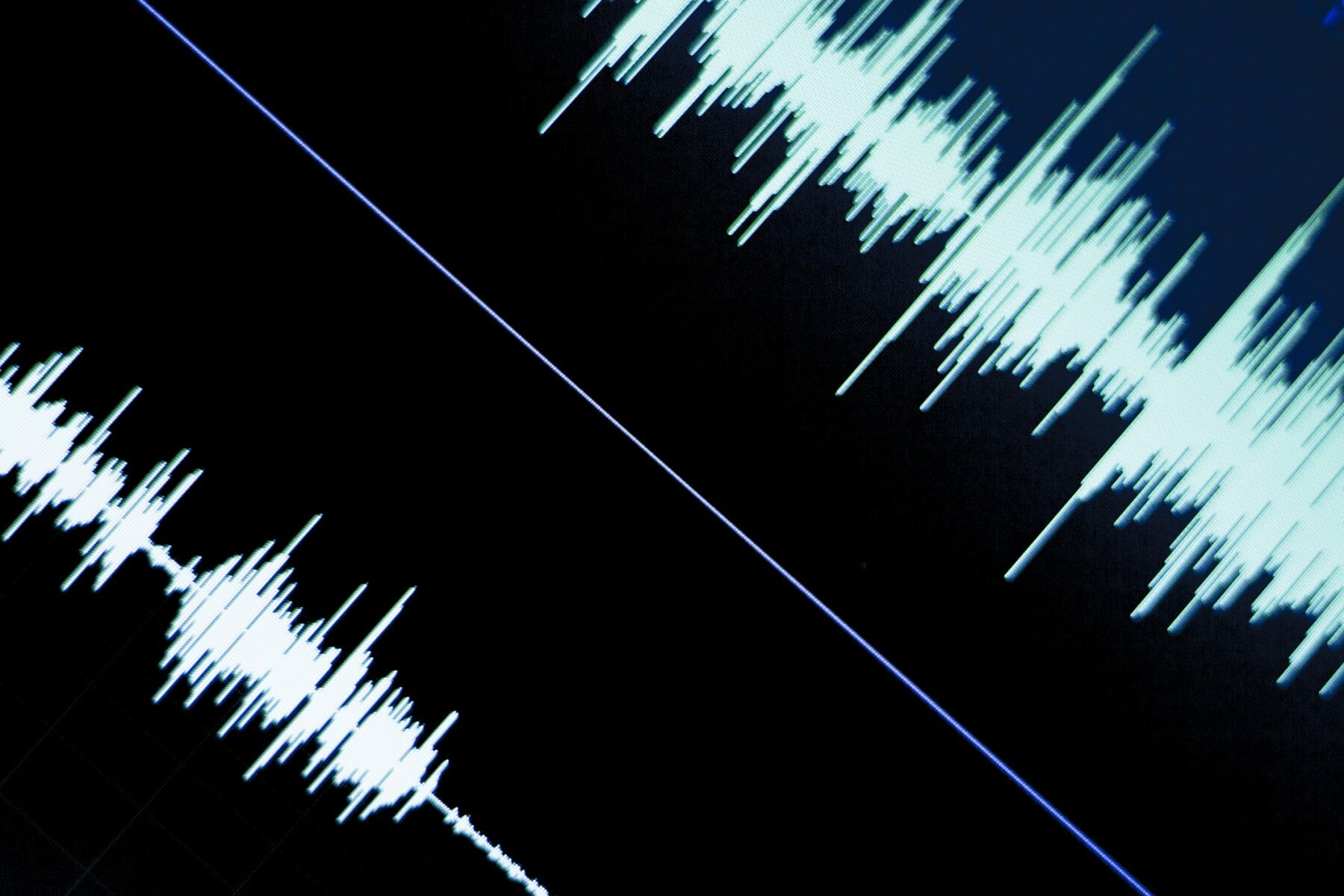
In December, New York City introduced a groundbreaking bill on algorithmic accountability. Once signed into law by Mayor Bill de Blasio later this year, the bill will establish a task force to investigate bias in algorithms and how these biases inform the work of city agencies and subsequently affect New Yorkers’ lives. The real question, however, is how algorithms that have quickly become pervasive across industries and the public sector hold the potential to discriminate against people based on their age, race, religion, gender, sexual orientation or citizenship status. The implications for the public sector, including the future of education, are significant.
Background on the Bill
The current bill came into being when New York City Council Member James Vacca read ProPublica’s investigation into racially biased algorithms and their impact on the criminal risk of defendants. What ProPublica‘s investigation revealed was algorithms are increasingly being used to calculate criminal risk but the algorithms aren’t as neutral as many people assume. Programmed by humans, these algorithms in fact perpetuate longstanding biases. The problem is that they are increasingly being used to inform decisions about who can be set free at every stage of the criminal justice system. And as the ProPublica article emphasizes, “Rating a defendant’s risk of future crime is often done in conjunction with an evaluation of a defendant’s rehabilitation needs. The Justice Department’s National Institute of Corrections now encourages the use of such combined assessments at every stage of the criminal justice process. And a landmark sentencing reform bill currently pending in Congress would mandate the use of such assessments in federal prisons.”
Moving forward, algorithms, not judges, will play an increasingly large role in deciding people’s futures. But the criminal justice system is not the only public sector realm increasingly leaning on algorithms.
From health care to K-12 and higher education, more and more public sector fields are embracing algorithmic solutions. In a large city, such as New York, one can naturally understand why algorithms are appealing. After all, how else can you process over 75,000 eight graders applying to over 400 high schools each year? Without algorithms, many ongoing tasks (e.g., placing kids in appropriate schools based on level, potential and district) would be nearly impossible. If, however, those algorithms are skewed, these efforts will be undermined and this is how the city’s innovative new bill came into being. As the bill’s sponsor, James Vacca, explains, “My ambition here is transparency, as well as accountability.”
Drafting a Bill to Address Bias in Algorithms
At its most basic, the proposed bill “would require the creation of a task force that provides recommendations on how information on agency automated decision systems may be shared with the public and how agencies may address instances where people are harmed by agency automated decision systems.” However, as explained in a committee report submitted in October 2017, the scope of the bill is also potentially more far-reaching. The report specifically flags the danger of using algorithms to make decisions about criminal justice situations.
Potential Impacts on Education
While the current concern about algorithms and bias primarily focuses on the criminal justice system, there is no question that as algorithms are increasingly used to create learner-centered experiences in schools and higher education, educators will also need to be aware of the potential ways in which algorithms may begin to reproduce biases in the school system. For example, if a student has historically under achieved on tests or worse yet, belongs to a demographic with a history of under achieving, there is the potential that these patterns may be used to reproduce rather than disrupt the pattern of under achievement in question.
3 Comments