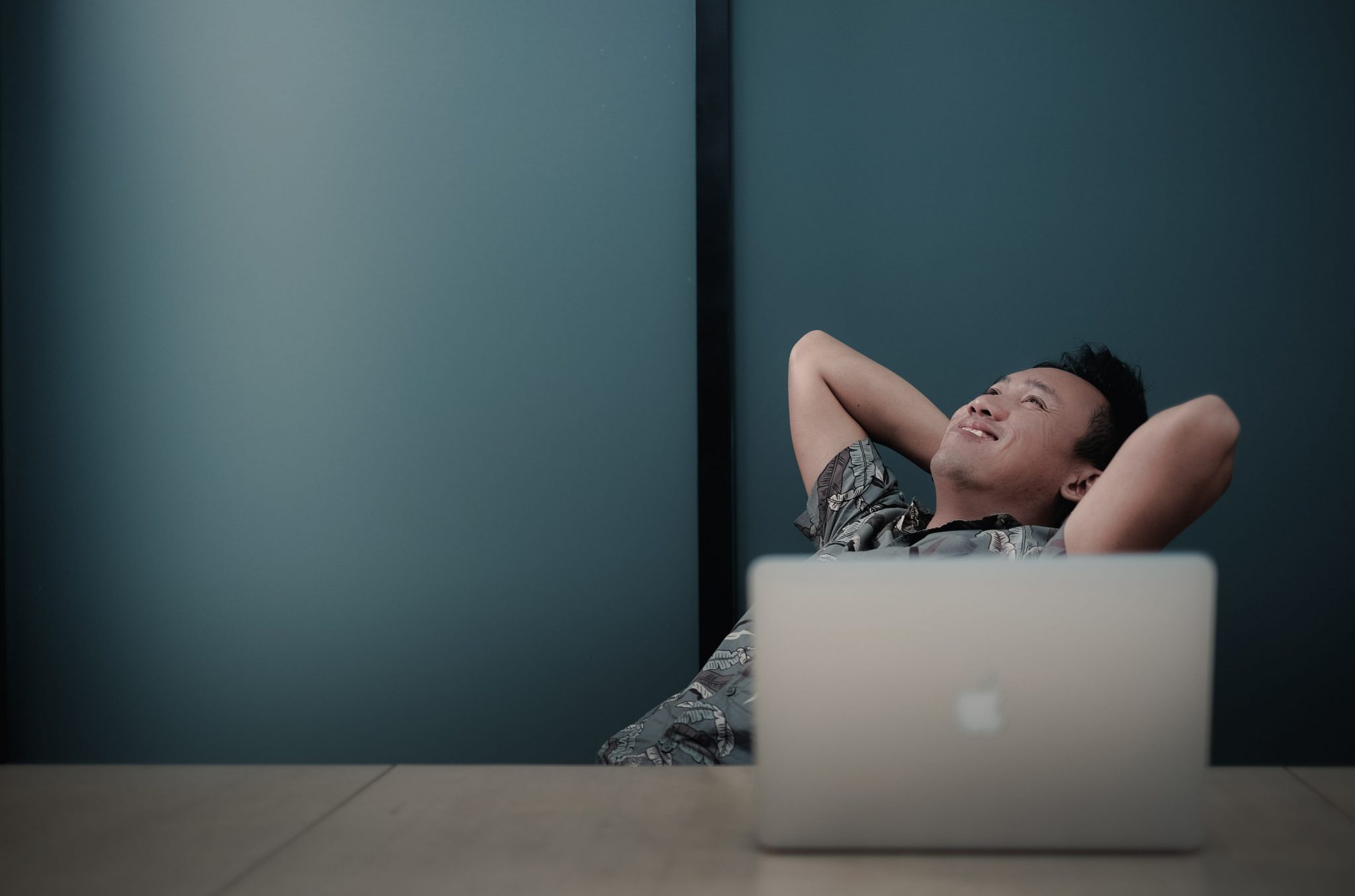
Articles
Editor’s Picks
Can You Measure Learner Engagement By Watching a Student’s Face?
By Henry Kronk
September 11, 2018
Measuring learner engagement in education is tantamount to assessment and efficacy. An engaged student intakes content easier, understands it better, and retains it longer. So, how do you know if a learner is engaged? Teachers who know their students can tell in a vague sense how a lesson went over. But with distance education, institutions might rely exclusively on student feedback. This method is less than ideal for numerous reasons. But what if there was another way? What if you could tell whether a learner is engaged just by watching their face? That is the question that researchers Mohammed Soleymani and Marcello Mortillaro from the University of Geneva sought to answer in a recent study.
With the advent of small, cheap, high resolution cameras along with adaptive algorithms, technologists from many fields dream of a way to track user interest. The use of such technology could revolutionize the way people learn. VIPKid, the Chinese tutoring giant, is already using its latest round of VC funding to track learner’s eye movement as a means to judge their engagement. The implications, however, could be much greater.
The Promise of Face-Tracking Technology
Imagine a personalized learning curriculum that could track down to the paragraph when and where a learner lost interest. It could completely change the way subjects are taught, how educational content is created, and ultimately, how effectively learners learn.
But before Soleymani and Mortillaro began to answer how one might begin to do this, they needed to determine if it’s possible in the first place. And the meager existing body of research doesn’t provide much optimism in that regard.
While humans tend to reflect basic emotions with more or less universal expressions, that of engagement or interest is more difficult to nail down. A few early studies conclude simply that one cannot measure learner engagement via facial expression. Others report accuracies of determination well below 50%.
Still, others have had much better success. One breakthrough came with a 2017 study in which Mortillaro participated which found that tracking the dynamic, moving motions of the face—compared to static images—increased accuracy from 29% to 68%.
In an effort to determine learner engagement, Soleymani and Mortillaro used three different methods. First, they mapped the face into nearly 50 different points and tracked their motion in relation to each other. Second, they looked at eye gaze duration and bodily posture. Third, they measured galvanic skin response (GSR), or the electric activity at the level of the skin. This method is used in everything from polygraphs to athletic fitness testing and involves attaching electrodes to numerous points on the body.
The researchers showed over 50 participants dozens of images and gifs. Participants were asked to rate each image and video on a scale from 1 to 7 based on interest. Soleymani and Mortillaro then used a Random Forest regressor to process the data, comparing their visual findings with the participants’ reported interest.
Measuring Learner Engagement
On a basic level, researchers found that they could indeed correlate learner engagement with a set of behaviors. Interested participants tended to smile, move their head closer to the screen, and among many other combinations, saccade their eyes, or move them rapidly between two points, for a longer than normal duration.
Some motions also indicated disinterest: “All participants leaned toward the screen when a new image appeared on the screen (attention toward a novel stimulus), but only when the stimulus was interesting they maintained the posture and remain engaged; when the stimulus was not interesting they would go back to the resting position, distancing themselves from the screen.”
Also of note, “micro-videos elicited more consistent behavioral patterns across participants, as is observable in the participant-independent results. We believe that the still images could not elicit emotions and reactions as strong as those elicited by moving pictures and therefore we suggest using videos in future work.”
Measuring interest and engagement via a learner’s face, therefore, may not be as far away as some think. Still, the authors conclude with a warning: “Obviously, using behavioral signals such as facial expression to detect situational interest requires capturing facial images and we should be aware that users might find that intrusive, for at least two reasons. First, users might not want to share information that would make them identifiable with a system. Second, one might not necessarily want to share his/her inner state such as interest in a given content. Deploying such systems should be only done with the full informed consent of its users and the users should have full control over how and where the data can be used. Such systems should be designed not to transfer or store identifiable information, in this case facial images. One existing solution is to execute facial tracking on users device and only transfer or store the analysis outcome.”
Featured Image: Ali Yahya, Unsplash.
[…] just because school is closed does not mean learning needs to stop. Using technology like learning management systems (LMS), video conferencing software, email, online libraries and databases, and more, instructors can […]