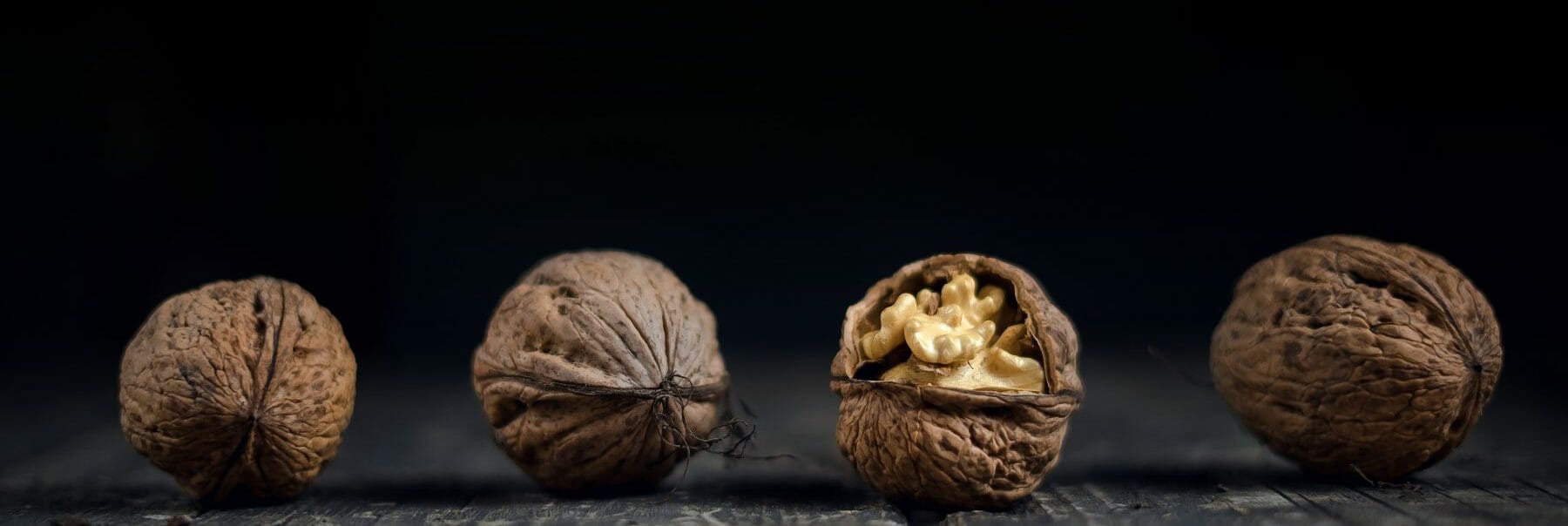
For years, student grades have been formed exclusively through how learners perform on certain assignments and criteria. In some subjects, like math, a student’s abilities may be very apparent. They will either be able to answer a given problem correctly or they will not. But in other areas, it’s foggier and much more subjective. Inconsistencies across grades and classes are inevitable. In a best case scenario, these inconsistencies are informed by human error or individual connections. In a worst case scenario, societal biases creep in and reinforce existing stereotypes among younger generations. Two researchers from Utrecht University in the Netherlands think they have a solution: expert elicitation.
Expert Elicitation
As authors Kimberley Lek and Rens Van De Schoot write, “Sometimes, experts possess unique knowledge, that is impossible or impractical to attain using traditional data collection methods. In those instances, expert elicitation can be used to “obtain” this knowledge. Specifically, the purpose of expert elicitation is to “construct a probability distribution that properly represents expert’s knowledge/uncertainty” (O’Hagan et al., 2006, p. 9), such that this expert knowledge can be used in—for instance—research, engineering projects and decision-making.”
Expert elicitation involves collecting a large body of expert opinion, quantifying it, and then delivering a conclusion based on this distributed data. The method is currently used widely in fields such as health, environmental research, and risk assessment.
In teaching, implicit bias is a well-established phenomenon. A 2015 study asked 16,000 high school teachers to predict each of their 10th graders’ future educational achievements. When the student in question was black, white teachers were about 30% less likely to predict they would get a college degree compared to black teachers.
As one of the authors, Seth Gershenson, wrote in a subsequent article for the Brookings Institution, “These results are not meant to, nor should they, demonize or implicate teachers. Biases in expectations are generally unintentional and are an artifact of how humans categorize complex information.”
A Digital Guard Against Bias
Lek and Schoot recognize expert elicitation as a way around this, but they also see many other potential benefits.
“An advantage of making these judgments explicit is that the elicitation tool can function as a feedback instrument for the teacher,” they write. “When used on multiple occasions, for instance, the teacher can see how his view on the child’s development has changed and he can evaluate what (rational and/or irrational) events have led to this change. Another advantage: when multiple teachers teach the same class, it is possible to quantitatively compare the judgments of these teachers, making differences in judgments directly apparent and open for discussion. Furthermore, the process of completing the elicitation tool can provide useful feedback as well. For example, when a teacher finds the elicitation difficult for a certain pupil, he knows that his view on this pupil’s development is still a bit vague.”
To study the use of expert elicitation, they developed software that would log students’ ability in a math course. To judge student ability in a given area, 24 teachers were asked to place their collective 504 students’ abilities among a range of 1-5, 1-10, 1-25, or 1-50, using puppets for units. If a child was given an assessment of 4 puppets out of 5, they’d be ranked roughly in the 80th percentile of their class.
As the authors write, “In order to obtain a distribution for every pupil, we also need to have an indication of the uncertainty of the teacher with regards to the positions chosen (i.e., the teachers’ judgment confidence). Obtaining such an estimate of uncertainty is a delicate matter, since people are known to generally underestimate their uncertainty (Lichtenstein et al., 1982; see also Bier, 2004; Speirs-Bridge et al., 2010). Additionally, most elicitation procedures ask the experts to state their uncertainty using precise probabilities (e.g., “90% certain”), something that is hard for people who are layman with respect to statistics. With the scales in Figure 1, however, obtaining an indication of uncertainty is rather intuitive and simple. Teachers simply choose the scale (Figures 1A–D) at which they feel certain enough to position their pupil(s). The scale with 5 “puppets,” for instance, is coarser than the scale with 25 “puppets,” and thus the teacher who chooses the latter scale is inherently more certain than the teacher who chooses the 1–5 scale. By using this approach to eliciting the teachers’ uncertainty, we avoid the necessity to ask for precise probabilities.”
The authors then used the software they created to graph teachers’ assessments. “In one glance,” they write, “the teacher can see his or her judgments (the peak of the distributions), how confident he/she is in these judgments (the width of the distribution) and how his/her judgments and judgment confidence differ over pupils. Now that it is visualized, these judgments can easily be shared with others, such as colleague teachers, the headmaster, parents, etc.”
Featured Image: Elijah O’Donnell, Unsplash.
No Comments